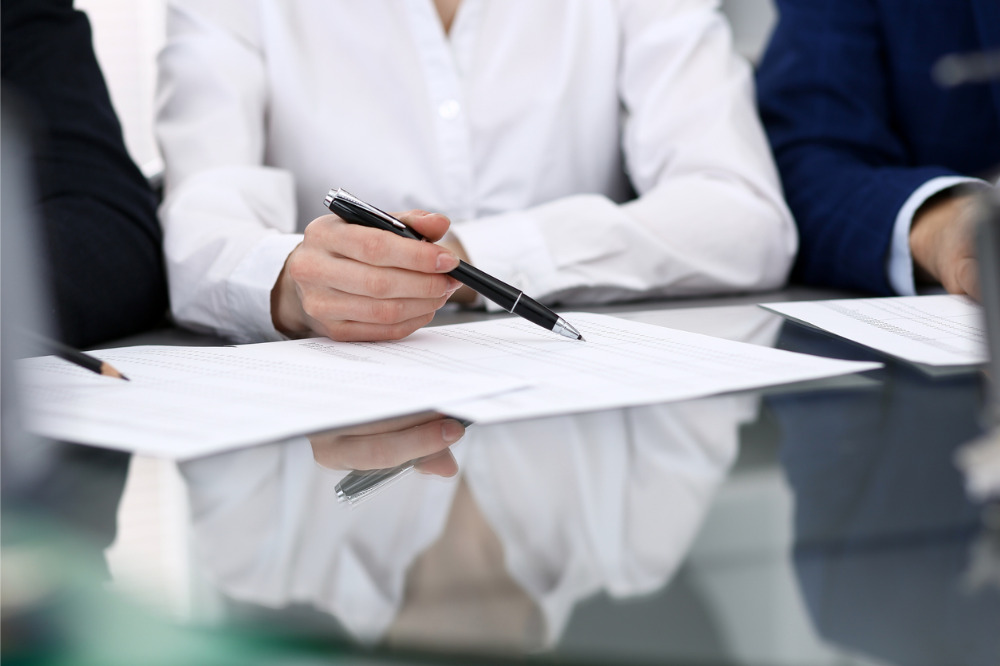
Large-scale international assessments such as PISA are not without flaws. No study is. As it is the case for science in general, large-scale assessments aim at describing and understanding student achievement and education as best as possible.
This attempt is surely not without limitations, warranting a balanced discussion of large-scale assessment results against the background of their potentials and limitations.
This is what science is and has been all about. Calling for entirely abandoning large-scale assessments every time a limitation of these studies gains attention is counterproductive, but seems like an easy fix.
Large-scale assessments are the best instrument currently available to gauge and compare what students around the world know and can do and provide powerful tools for informing evidence-based policy decisions.
Their use goes far beyond the notorious league tables, and they help policymakers to identify potential for improvement, for instance, by investigating whether students from a lower socio-economic background learn as much as students that are better off.
A lot of research that combines expertise and state-of-the-art approaches from various fields goes into best practices for ensuring the validity of conclusions drawn from large-scale assessment data.
The instruments employed in large-scale assessments have been extensively studied by researchers around the world. An online search for the term “Test-Curriculum Matching Analysis” will reveal what TIMSS and PIRLS do to ensure that what is tested in these international assessments is relevant and taught in schools around the world.
Performance in large-scale assessments shows relationships to relevant variables such as class repetition, completion of high school, taking up tertiary education, or (future) income. This indicates that we are far from capturing only random noise with large-scale assessments, but rather relevant aspects for later success in life.
The results of large-scale-assessments may indeed reflect not only differences in achievement, but also other aspects to some extent, such as test-taking effort. There is a comprehensive amount of research on approaches for identifying non-effortful responding, and there have been repeated calls to make studies of these types of behaviours part of the reporting.
We believe that reporting findings from such studies in addition to differences in achievement would come with many advantages. We recently reported on how such enhanced reporting could look like under the title “Reframing Rankings in Educational Assessments” in Science.
Focusing not only on how many items students solve, but also on how they solve them would increase transparency by reminding readers of what large-scale assessments can and what they cannot provide.
Maybe even more important, we may shift the perspective on test-taking effort from seeing it as a mere nuisance that distorts data quality to embracing it as an additional source of information on the reasons for the observed performance on the tasks.
Such a shift in perspectives might support disentangling to what extent students may score low on a test due to lack of knowledge or due to lack of effort, or some other reasons such as time management, distractions, or differences in test-taking strategies.
These aspects may be of relevance for real-life outcomes, such as later educational attainment. At the same time, different sources of low performance may entail different types of educational interventions.
Hence, reporting on key aspects of test-taking behaviour alongside the performance that students showed may provide an ever-richer description of not only what students know and can do but also of how they do it. This can facilitate a better understanding of differences in performance and deriving appropriate interventions from large-scale assessment results.
Dr. Esther Ulitzsch is a Research Associate at the Leibniz Institute for Science and Mathematics Education in Kiel, Germany. She holds a PhD from Freie Universität Berlin. Her main research interests are the development of methods that make use of process data for modeling and understanding test-taking behavior in large-scale assessments.
Dr. Steffi Pohl is a Full Professor for Methods and Evaluation/Quality Assurance at the department of education and psychology at the Freie Universität Berlin, Germany. In the past, she has been responsible for analyzing the competence data of the German National Educational Panel Study. In her research, she develops statistical models for modeling and investigating test-taking behavior and evaluates its impact on results of competence assessments.
Dr. Matthias von Davier is the J. Donald Monan, S.J., Professor in Education at the Lynch School of Education and Human Development at Boston College. He served as senior research director for the analysis of PISA and PIAAC at Educational Testing Service, in Princeton, NJ, until 2016, and currently serves as executive director for the TIMSS & PIRLS International Study Center at Boston College.